Mouton, N. & Henrico, A.
Ensovoort, volume 44 (2023), number 11: 1
Abstract
The Covid-19 pandemic initially turned our world upside down, changing our perceptions on many issues in life. From a pedagogical perspective, it impacted and encouraged a new way of thinking about teaching and learning in the higher education environment. The abrupt enforcement of the lockdown in South Africa in March 2020 had a ripple effect on perceptions from now on concerning teaching and learning. At that stage, students in the asynchronous mode of delivery were perceived as the least affected when all teaching and learning converted fully to the online environment for all modes of delivery. As Covid-19 restrictions in South Africa eased down, the face-to-face modes of delivery are again being implemented across all South African universities. In hindsight, the question posed to asynchronous students during the post-Covid era was to reflect on their learning experiences during the Covid period of 2020-2022. This study is embedded in the positivist paradigm using a quantitative approach to collect and analyse data. This approach made it possible to objectively pinpoint and understand the (new) social world of asynchronous students according to predetermined theoretically-based variables. Probability sampling identified a representative sample of respondents. The results indicate how teaching and learning must be adapted to accommodate a new way of teaching and learning for postgraduate business school students in the asynchronous mode of delivery.
Keywords: asynchronous students, hybrid learning, technology, teaching and learning, higher education, online learning
Introduction
The Covid-19 pandemic compelled higher education institutions to adopt online strategies more strategically, impacting both synchronous and asynchronous modes of delivery. Student experiences rapidly shifted from their traditional perceptions of asynchronous education to a more streamlined model, which also influenced how lecturers viewed and approached both contact and asynchronous cohort students. While the concept of asynchronous education is not new, Covid-19 exposed shortcomings in the model, leading to immediate changes and adaptations to better cater to the needs of asynchronous students.
The overarching term “asynchronous education” encompasses adjunct, mixed, and online modes of delivery (Harasim, 2000). Perceptions of teaching and learning underwent a radical transformation in the post-Covid-19 environment as students and lecturers faced sudden changes, shifting from a world with other educational options to one where online education had to be embraced globally. Self-directed learning, flexibility in learning styles, and the idea of learning anytime and anywhere were all put to the test (Stewart & Lowenthal, 2021). Online learning became a more equitable space, leveling the playing field for all, regardless of their backgrounds or circumstances.
For the purposes of this article, asynchronous education is defined as partially offered online, where lecturers and students are separated in both time and space (Keegan, 2002).
Contextual Background
This reflective study was conducted in 2022 at a higher education institution, specifically for a module offered to postgraduate students at a business school. This module was mandatory for all enrolled students within this particular postgraduate program. It is structured as a semester-long module, spanning from February to July each year. Students enrolled in this program hail from various regions across the country, encompassing both rural and urban areas.
During the Covid-19 pandemic, students did not physically attend any on-campus classes. This aspect made the transition to online learning during Covid-19 somewhat smoother for them. It is a prerequisite for these students to possess computer literacy and access to the internet, thus facilitating their adaptability to the online learning environment.
Moreover, it is worth mentioning that a significant proportion of these students are working adults, implying that they generally possess a higher degree of maturity and experience compared to the traditional first-year students, who typically fall within the age range of 18 to 22 years old.
Problem Statement
In the post-Covid-19 era, students have adapted to a significantly altered learning environment. Prior to the pandemic, face-to-face teaching and learning were the preferred methods, and even students in the asynchronous mode organized in-person meetings for collaborative brainstorming and knowledge sharing. However, the Covid-19 pandemic wrought substantial changes in how both lecturers and students perceived the process of teaching and learning.
As we transition away from the Covid-19 era, a new educational landscape is emerging, and we find ourselves grappling with uncertainty regarding the future of teaching and learning. The current challenge revolves around understanding how students’ perspectives were influenced during the Covid-19 period and how these viewpoints have evolved as we move forward.
Research Questions
Research questions that impact the perceptions of students are formulated as follows:
- How do students perceive the availability of technology and software on the learning management site (LMS) of the university?
- How do students experience asynchronous education?
Literature Review
The literature will focus on the perceptions and challenges of students registered in the distance mode of delivery during the Covid-19 era. The focus will be on the impact of technology, the role of the LMS, e-resources and assessment methods. The role of the lecturer will also be scrutinised as perceived from the student’s point of view.
Asynchronous Education and the Changing Environment
Asynchronous education has a historical lineage dating back to the 1830s when it was initially conducted through postal correspondence (Bower & Hardy, 2004). It has since evolved through various stages, including satellite broadcasting (Harasim, 2000), telematic network courses (Moore & Kearsley, 2012), and has now found its place in the internet age.
Before the Covid-19 pandemic, several factors such as social presence, effective asynchronous communication, online student-student interaction, and the potential for asynchronous students to experience feelings of loneliness were mitigated by the introduction of a more user-friendly online and virtual mode of delivery (Stewart & Lowenthal, 2021).
Students who opt for the asynchronous mode of delivery are typically considered pro-asynchronous and are well-informed about the expectations and dynamics of this mode. However, it is crucial, during the development of such programs, to assess whether students are receptive to online courses (Fidalgo, 2022). Assumptions are often made that these students will feel at ease in the asynchronous mode and grasp the impact of technology on their learning experience. Therefore, it is generally presumed that these learners have made a conscious decision to move away from the traditional classroom approach and are fully aware of the implications of their choice to study in the asynchronous mode of delivery.
Students often gravitate towards asynchronous education because it offers self-pacing to some degree, flexibility in terms of time and space, time-saving benefits, and cost-effectiveness (Fidalgo et al., 2022). It is also commonly assumed that the quality of programs in the asynchronous mode is on par with or possibly even superior to that of traditional face-to-face delivery. However, it’s important to note that, especially in the pre-Covid-19 era, researchers have identified disadvantages, including feelings of isolation, challenges in maintaining motivation, a sense of detachment, and a lack of social interaction with peers (Fidalgo et al., 2022; De Paepe, Zhu, & Depryck, 2018; Lei & Gupta, 2010).
Significantly, research conducted by Horspool and Lange (2012) indicates that students often choose online programs to avoid scheduling conflicts, travel requirements, and other time-related constraints.
Theoretical Framework
The Covid-19 pandemic had a profound impact on the landscape of teaching and learning, necessitating the implementation of various techniques for virtual lecturing (Simamora, 2020). This effect was felt on a global scale, with online learning becoming a fundamental means of continuing education (Junus, 2021).
The rapid pace of technological change has led to shifting perspectives on how students perceive the learning process. Covid-19 further influenced these perceptions, resulting in changes both before and after the pandemic. Consequently, technology cannot be viewed in isolation but should be integrated with various technological tools and involve lecturers (Ellis et al., 2016). The adoption and utilisation of information technology in the workplace remain a significant concern within information systems practice, as the effective operation of hardware and software capabilities continues to affect the “productivity paradox” resulting from organisational investments in information technology (Venkatesh & Davis, 2000).
Hence, it is considered essential to adopt a supportive theoretical framework to introduce new technologies effectively. The Technology Acceptance Model (TAM) has become crucial for analysing factors that could impact human behavior (Muhaimin et al., 2019). TAM primarily focuses on two key elements: perceived usefulness (PU) and perceived ease of use (PEOU). Users’ attitudes towards technology can be predicted by their perception of how easy it is to use (Nigam, 2017). Davis explains that PU refers to the extent to which an individual believes that using a specific system would enhance their job performance.
In contrast, PEOU is based on the belief that using a particular system would be straightforward and hassle-free (Davis, 1989). TAM2, an extension of the original TAM, includes additional factors such as social influences (subjective norm, voluntariness, and image) and cognitive instrumental processes (job relevance, output quality, result demonstrability, and perceived ease of use) that can influence technology adoption behavior (Venkatesh & Davis, 2000). These factors are crucial as they reflect the impact of subjective norms, voluntariness, and image. Individuals often respond to social normative influences to establish a desirable image within their reference group, indicating that the subjective norm positively influences their image if a social group at work believes that certain behaviors should be performed (Venkatesh & Davis, 2000).
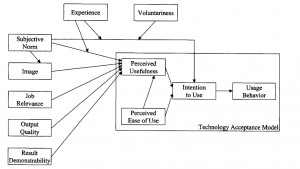
Online Learning
Online learning, as defined by Elfaki et al. (2019), encompasses the use of electronic technologies to access curriculum content outside the traditional face-to-face classroom setting. In higher education, it is often associated with programs that are fully delivered online, although a blended approach, which combines online activities with in-person instruction, is also common (Crawford, 2014). The primary objective remains the enhancement of teaching quality and bridging the gap between lecturers and students (Lee, 2017). Online Learning can take various forms, including synchronous, asynchronous, and hybrid modes of instruction. However, effective communication and feedback to students remain integral components regardless of the specific mode.
Learning Management Systems (LMS)
LMS has gained significant importance, particularly in asynchronous education within higher education institutions (HEIs) (Abdallah et al., 2019; Alenzi, 2018). It functions as a web-based platform for managing, documenting, reporting, and sharing educational resources (Ghilay, 2017). LMS has contributed significantly to facilitating teaching and learning by creating a collaborative, personalised, and flexible learning environment (Mershad et al., 2020). It empowers students to engage in independent, self-regulated virtual learning experiences (Maphalala & Adigun, 2020). Moreover, LMS allows for the sharing of pedagogical content and student experiences across geographical boundaries, reducing disparities and accommodating diversity (Cross, 2022). Additionally, it provides a platform to administer, deliver, and track students’ progress, thereby enhancing participation and skills for both lecturers and students (Pandey, 2020).
Learning Resources
The development of learning resources forms the foundation of asynchronous education activities. According to Li et al. (2021), there is often uneven quality in these resources, and their blanket application may lack effective management, leading to disorganised resources that hinder their utilisation and effective sharing. The sharing of asynchronous education resources is gaining increasing importance, with traditional boundaries being dismantled, allowing for the sharing of teaching tools, equipment, and educational information among lecturers (Li et al., 2021).
Assessment in Asynchronous Learning
Assessing student learning in asynchronous education can be challenging due to the absence of real-time, face-to-face interaction between instructors and students (Moore & Kearsley, 2005). In traditional classrooms, instructors can gauge student understanding through active participation, in-class quizzes, exams, and assignments. In contrast, distance learning relies on alternative assessment methods. Some of these methods include:
- Online quizzes and exams: These assess students’ knowledge of course content.
- Assignments: To evaluate students’ ability to apply concepts learned.
- Projects: To assess critical thinking and problem-solving skills.
- Discussion forums: To gauge participation and the ability to communicate ideas.
- Peer assessment: Allows students to provide feedback on each other’s work.
Challenges in Assessing Student Learning in the Asynchronous Mode of Delivery
It is important that we also examine the challenges that students face in an asynchronous environment.
Lack of Direct Interaction
In a post-Covid environment, students often face a notable absence of face-to-face interactions with peers and instructors. This shift challenges their ability to engage in spontaneous discussions, collaborative projects, and the development of social connections within the educational context.
Instructors Cannot Observe Students in Real Time
With the transition to remote learning, instructors lose the ability to observe students’ reactions and comprehension in real-time. This impedes their capacity to gauge student engagement, adjust teaching methods accordingly, and provide timely feedback for effective learning outcomes.
Technical Difficulties
The post-Covid educational landscape has highlighted the prevalence of technical difficulties as a significant hurdle for students. Issues such as software glitches, hardware malfunctions, and compatibility problems can disrupt the learning process and cause frustration for both students and instructors.
Internet Connectivity Issues May Disrupt Assessments
Reliable internet connectivity is essential for remote assessments, yet many students encounter connectivity challenges. This poses a risk to the integrity of online assessments, potentially affecting the fairness and accuracy of students’ evaluations in a post-pandemic teaching environment.
Lack of Social Interaction
Students may miss out on peer and instructor interaction, affecting motivation. To address these challenges, lecturers must use a variety of assessment methods, provide clear instructions, offer timely feedback, and remain flexible and understanding of students’ unique circumstances in asynchronous learning. The choice of assessment methods should align with course objectives and ensure fair and accurate evaluation of student learning.
Research Methodology
A cross-sectional quantitative research design was employed, involving a data collection instrument, statistical analysis, and assessment of statistical significance to investigate the relationships between variables identified in the literature (Bryman et al., 2014:106). Quantitative data allowed for a systematic and standardised measurement of variations and the establishment of a consistent benchmark.
Data Collection, Population and Sampling
The target population for this study was selected using convenience sampling, focusing on students enrolled in a specific asynchronous semester module. Initially, the population comprised 385 students, and the study aimed to collect responses from at least 20% of the population, resulting in an N of 83 (23%), deemed sufficient for reliable results. A cross-sectional study design and a single-stage sampling method were employed for ease of participant selection. Survey questions were structured using a Likert scale ranging from 1 to 5, with 1 denoting “Strongly Disagree” and 5 indicating “Strongly Agree.”
The survey encompassed 33 statements organised into two sections. Section 1 included demographic questions, while Section 2 consisted of five parts:
- Learning Management System (4 questions).
- Perceptions of asynchronous education (7 questions).
- The role of the lecturer (12 questions).
- Technology and its perceived usefulness (4 questions).
- Experiences of assessment (5 questions).
Research Procedure
All 385 students enrolled in the asynchronous module were invited to participate by completing an electronic questionnaire. A consent letter was distributed via the online platform, explaining the study’s purpose and objectives. Respondents were given a three-week window to complete the questionnaire, with weekly reminders sent to encourage participation and maximise response rates. Ethical procedures, in accordance with the institution’s policy and Covid-19 protocols, were adhered to throughout the process.
Data Collection Instrument
The data collection instrument for this quantitative study was a meticulously designed survey, the primary tool for gathering structured information. Comprising well-crafted questions, the survey utilised a Likert scale and multiple-choice format to collect quantitative data, ensuring precise measurement and analysis. The survey covered key variables, including demographics, perceptions, and attitudes, aligning with the research objectives. Rigorous testing was conducted to ensure clarity, consistency, and reliability. The instrument was administered electronically, combining participant convenience with data integrity.
Data Analysis
Data analysis encompassed several key steps. Firstly, a data summary was transferred from Google Sheets to Excel for initial processing. Subsequently, statistical analysis was conducted using the IBM Statistical Package for the Social Sciences (IBM SPSS). This software facilitated the validation of initial research assumptions and the extraction of conclusions based on identified data trends (IBM, 2019:1).
Scores were assigned to each variable by aggregating values from different sections of the data, allowing for the calculation of means and standard deviations. IBM SPSS’s analytical capabilities played a crucial role in uncovering patterns, relationships, and trends within the dataset, ensuring research findings’ reliability and validity.
Results
Demographics
This section focussed on the demographical information, which included three variables, viz., the age, work experience and highest qualifications of the participants (Table 1). These were considered the only demographical information that could influence the other variables.
VARIABLES | FREQUENCY | % | |
Age | 21-25 | 11 | 13,3 |
26-35 | 47 | 56,6 | |
36-45 | 21 | 25,3 | |
46-55 | 4 | 4,8 | |
Work experience | 0-3y | 17 | 20,5 |
4-10y | 38 | 45,8 | |
11-15y | 15 | 18,1 | |
16-20 y | 9 | 10,8 | |
21 y or more | 4 | 4,8 | |
Highest qualification | Less than Gr 12 | 0 | 0 |
Gr 12 | 0 | 0 | |
Post school diploma or equivalent | 5 | 6,0 | |
Degree or equivalent | 64 | 77,1 | |
Honours | 11 | 13,3 | |
Masters | 2 | 2,4 | |
PhD | 1 | 1,2 |
Table 1: Demographics of students (N=83)
The study revealed that a majority of the respondents fell within the age range of 26-35, possessed 4-10 years of work experience, and held at least a degree or an equivalent qualification. This observation aligns with a study conducted by Henrico and Mouton (2021), which also noted a similar trend. It is worth mentioning that Henrico and Mouton’s study focused on the pre-Covid-19 era, and this research produced consistent findings despite the pandemic’s impact. The admission criteria for the postgraduate program specify that students must hold a degree or an equivalent qualification. It is important to note that some respondents may have qualified through Recognition of Prior Learning (RPL). Consequently, the survey included questions to determine whether respondents possessed less than a Grade 12 qualification or at least a Grade 12 qualification, considering the possibility of RPL admissions. Additionally, the data revealed that 13.3% of the respondents (11 individuals) held an Honours degree, 2.4% (2 respondents) had a master’s degree, and 1.2% (1 respondent) held a PhD. This distribution suggests that the respondents were a mature and experienced group, with significant educational qualifications and work experience, which may have influenced their perceptions of teaching and learning in a post-Covid-19 educational landscape.
Data validity and reliability
In our study, we employed the Kaiser-Meyer-Olkin (KMO) measure of sampling adequacy to assess the suitability of our data for factor analysis, a common method for reducing data complexity. The KMO values obtained ranged from 0.63 to 0.93 for each section of the questionnaire (see Table 2), indicating that our data were well-suited for this analysis. Generally, KMO values above 0.6 are considered acceptable for factor analysis, as recommended by Kaiser (1970, 1974).
Furthermore, we conducted Bartlett’s test of sphericity, which assesses whether the correlation matrix is significantly different from an identity matrix. In our case, Bartlett’s test yielded a highly significant p-value of 0.000, well below the conventional significance threshold of 0.05. This outcome affirms that our variables were indeed interrelated and that our correlation matrix was suitable for factor analysis.
Based on these robust validation results, we confidently retained the six factors derived from our principal component exploratory factor analysis, as detailed in Table 2. This process ensures the reliability and validity of our inferential statistical analysis method for investigating the relationships between these factors in our study.
Table 2: Validity and Reliability Tests
FACTORS | VALIDITY | RELIABILITY |
---|---|---|
KMO | Total % variance explained | |
Availability of technology | 0.64 | 75.79 |
Respondents’ views on asynchronous education | 0.83 | 63.99 |
The role of the lecturer | 0.93 | 67.31 |
Availability of software resource on the LMS | 0.82 | 80.12 |
Types of assessment | 0.87 | 77.48 |
Asynchronous mode of delivery | 0.63 | 0.00 |
Factor 1: Availability of Technology
This factor achieved a KMO value of 0.64, indicating moderate sampling adequacy. It accounts for 75.79% of the total variance, and its internal reliability is high, with a Cronbach’s Alpha coefficient of ≥ 0.8.
Factor 2: Respondents’ Views on Asynchronous Education
This factor demonstrated a strong KMO value of 0.83, explaining 63.99% of the total variance. It also exhibits excellent internal reliability, with a Cronbach’s alpha coefficient of ≥ 0.8.
Factor 3: The Role of the Lecturer
This factor shows a very high KMO value of 0.93, explaining 67.31% of the total variance. Its internal reliability is excellent, with a Cronbach’s alpha coefficient of ≥ 0.8.
Factor 4: Availability of Software Resources on the LMS
This factor yielded a robust KMO value of 0.82, explaining 80.12% of the total variance. Its internal reliability is high, with a Cronbach’s alpha coefficient of ≥ 0.8.
Factor 5: Types of Assessment
This factor achieved a strong KMO value of 0.87, explaining 77.48% of the total variance. Its internal reliability is excellent, with a Cronbach’s alpha coefficient of ≥ 0.8.
Factor 6: Asynchronous Mode of Delivery
This factor exhibited a KMO value of 0.63, indicating moderate sampling adequacy. However, it does not explain significant variance (0.00%), suggesting that this factor may require further refinement or examination.
Overall, the high Cronbach’s Alpha coefficients for the first five factors (≥ 0.8) indicate strong internal reliability, suggesting that the survey questions within these factors are consistent and reliable in measuring the intended constructs. Additionally, the substantial variance explained by these factors underscores their relevance and contribution to the study.
Descriptive Statistics
The questionnaire comprised six distinct sections designed to elicit respondents’ perspectives. We conducted a descriptive analysis of both independent and dependent variables. Table 2 offers a summary of the six additional variables employed in this study. A systematic procedure identified six factors aimed at gauging respondents’ perceptions of asynchronous delivery mode.
These factors are explained as follows:
Factor 1: Availability of Technology
This section included three questions to assess whether respondents had access to the necessary technology, data, and internet connectivity required for effective engagement with teaching and learning challenges.
Factor 2: Respondents’ Views on Asynchronous Education
This section encompassed seven questions to discern respondents’ preferences regarding asynchronous or traditional contact education, exploring their perspectives, readiness, technical and academic preparedness, and comfort level with virtual peer interactions.
Factor 3: The Role of the Lecturer in an Asynchronous Learning Environment
The lecturer’s competence, familiarity with the mode, attentiveness to technological challenges, and ability to engage virtually are critical. To evaluate this, twelve questions were used to ascertain the role of the lecturer.
Factor 4: Availability of Software Resources on the LMS
This section consisted of three questions, aiming to determine whether respondents could access resources in a timely manner and whether these resources enhanced their learning experience.
Factor 5: Types of Assessment
This section featured five questions delving into the significance of assessments and their practical applicability in the workplace, including varied assessment structures and the role of communication and discussion forums.
Factor 6: Asynchronous Mode of Delivery
Factor 6 scrutinises the effectiveness and impact of asynchronous learning, considering flexibility, engagement, and suitability for various learning styles.
Outcomes of the Study
Based on the statistical information, it is also necessary to link the objectives of the study with the outcomes of the factors.
The first objective (How do students perceive the availability of technology and software on the learning management site (LMS) of the university?), links with Factors 1 (Availability of Technology) and Factor 4 (Availability of Software Resources on the LMS) whereas the second objective (How do these students experience asynchronous education?), links with Factors 2 (Respondents’ Views on Asynchronous Education ), 3 (The Role of the Lecturer in an asynchronous learning environment), 5 (Types of Assessment) and 6 (Asynchronous Mode of Delivery).
The outcomes of the study will be discussed based on the objectives and factors that were analysed:
Factor 1: Availability of Technology
The assessment of the Availability of Technology factor indicates that respondents in this study generally possess the requisite technological resources, including access to technology, data, and internet connectivity, necessary for effective engagement with teaching and learning challenges. This finding is supported by a high Cronbach’s alpha coefficient (≥ 0.8), reflecting strong internal reliability within this factor.
Factor 2: Respondents’ Views on Asynchronous Education
Regarding respondents’ views on asynchronous education, they highly value the role of the lecturer (correlation coefficient .764) in facilitating online teaching and learning. This importance extends to various assessment types offered in the module (correlation coefficient .765). The availability of software resources also proves critical, affecting technology access, perceptions of asynchronous education, assessment methods, and overall views on teaching and learning in the asynchronous mode of delivery.
Factor 3: The Role of the Lecturer in an Asynchronous Learning Environment
The above is characterised by a very high KMO value of 0.93 and explains 67.31% of the total variance, underscoring its significance. The factor demonstrates excellent internal reliability with a Cronbach’s alpha coefficient of ≥ 0.8. These results affirm the pivotal role of the lecturer in asynchronous learning and emphasise the factor’s relevance in understanding the dynamics of modern education.
Factor 4: Availability of Software Resources on the LMS
“Availability of Software Resources on the LMS,” exhibited a robust KMO value of 0.82 and accounted for 80.12% of the total variance, indicating its central role. With a Cronbach’s alpha coefficient of ≥ 0.8, this factor signifies the critical importance of software resources within the Learning Management System, underscoring their role in facilitating effective education.
Factor 5: Types of Assessment
Types of Assessment,” displayed a strong KMO value of 0.87 and explained 77.48% of the total variance, highlighting its substantial influence. Its Cronbach’s alpha coefficient, ≥ 0.8, confirms its internal reliability. These findings underscore the significance of varied assessment methods in the educational landscape, emphasising their role in comprehensive learning experiences.
Factor 6: Asynchronous Mode of Delivery
Asynchronous Mode of Delivery, exhibited a moderate KMO value of 0.63, indicating room for improvement in factor clarity. However, it explained 0.00% of the total variance, suggesting limited relevance. Further refinement or investigation may be needed to better understand the role and impact of asynchronous delivery in this educational context.
Limitations and Future Research
This study provides valuable insights tailored primarily to postgraduate lecturers within specific institutions and those sharing similar characteristics. However, the generalisability of findings is constrained by the relatively small participant pool. Given the marked resource disparities across regions in our developing country, it is advisable to apply these outcomes cautiously, particularly within higher education institutions (HEIs) situated in comparable regions.
Future research endeavors should encompass a broader spectrum of HEIs, including those with more resources, and those offering postgraduate programs or equivalent qualifications. This comparative analysis can further enrich our understanding of HE dynamics, facilitating more extensive knowledge application in the higher education landscape moving forward.
Conclusion
In conclusion, this study highlights the critical role of adopting a robust theoretical framework for the successful integration of new technologies into the educational landscape. The Technology Acceptance Model (TAM) and its extension, TAM2, emerge as invaluable tools in this context. TAM’s focus on perceived usefulness (PU) and perceived ease of use (PEOU) offers valuable insights into the factors shaping user attitudes toward technology adoption. Additionally, TAM2, encompassing social influences and cognitive instrumental processes, provides a comprehensive framework for analysing the multifaceted dynamics of technology acceptance and integration.
As depicted in Figure 1, these models offer a structured approach to understanding the intricate interplay of factors influencing technology adoption, particularly in the post-pandemic educational environment. This research concludes that respondents exhibit confidence in their ability to excel in the asynchronous mode of delivery. Factors such as technology availability, the lecturer’s role, assessment methods, and resource accessibility contribute significantly to their positive learning experiences in this mode. These findings underscore the relevance of TAM and TAM2 as indispensable tools for researchers and lecturers navigating the evolving landscape of technology-enhanced education.
References
Abdallah, N.A., Ahlan, A.R. & Abdullah, O.A. 2019. The role of quality factors on learning management systems adoption from instructors’perspectives. The online journal of asynchronous education and e-learning, 7(2):133.
Adigun, O.T. 2020. Computer-assisted instruction, project based learning and achievement of Deaf learners in Biology. Journal of e-Learning and Knowledge Society, 16(1):23-32.
Alenezi, A. 2017. Obstacles for teachers to integrate technology with instruction. Educational Information Technology 22:1797–1816. https://doi.org/10.1007/s10639-016-9518-5 (Accessed on 23 March 2022).
Cross, J.A. 2021. Implementing E-Learning. Lance Dublin: American Society for Training and Development.
Davis, F.D. 1989. Perceived usefulness, perceived ease of use, and user acceptance of information technology. MIS quarterly:319-340.
De Paepe, L., Zhu, C., & Depryck, K. (2018). Online Dutch L2 learning in adult education: Lecturers’ and providers’ viewpoints on needs, advantages and disadvantages. Open Learning,33(1), 18–33.https://doi.org/10.1080/02680513.2017.1414586.(Access on 3 September 2022).
Dudovskiy, J. 2018. The ultimate guide to writing a dissertation in business studies: A step by step assistance [eBook]. Published by research-methodology. net.
Elfaki, N.K., Abdulraheem, I. & Abdulrahim, R. 2019. Impact of e-learning vs traditional learning on student’s performance and attitude. International Journal of Medical Research & Health Sciences, 8(10):76-82.
Field, A. 2017. Discovering statistics using IBM SPSS. 5th ed. London, UK: Sage.
Fidalgo, P., Thormann, J.; Kulyk, O. & Lencastre, J.A., 2020. Students perceptions on asynchronous education: A multinational study. International Journal of Educational Technology in Higher Education, https://educationaltechnologyjournal.springeropen.com/articles/10.1186/s41239-020-00194-2Ghilay, Y. 2017. Online learning in higher education. Online Learning in Higher Education. New-York: Nova Science Publishers. (Accessed on 6 October 2022).
Horspool, A., & Lange, C. 2012. Applying the scholarship of teaching and learning: Student perceptions, behaviours and success online and face-to-face. Assessment & Evaluation in Higher Education, 17(1), 73–88 Retrieved from https://srhe.tandfonline.com/doi/abs/10.1080/02602938.2010.496532#.XX-XPigzZPY (Accessed on 20 September 2022).
Keegan, D. 2002. The future of learning: From eLearning to mLearning. Hagen: Zentrales Institut fur Fern Universitat Retrieved from https://www.academia.edu/3442041/The_future_of_learning_From_eLearning_to_mLearning (Accessed on 3 August 2022).
Junus, K., Santoso, H.B., Putra, P.O.H., Gandhi, A. and Siswantining, T. 2021. Lecturer readiness for online classes during the pandemic: A survey research. Education sciences, 11(3):139 – 153.
Lee, K. 2017. Rethinking the accessibility of online higher education: A historical review. The Internet and Higher Education, 33:15-23.
Li, C., Zhao,Q., Herencsar, N. &Srivastave, G. 2021. The Design of Mobile Asynchronous Online Education Resource Sharing from the Perspective of Man-Machine Cooperation. Mobile Networks and Applications. 26:2141-2152.
Mershad K, Damaj A, Wakim P & Hamieh A. 2020. LearnSmart: A framework for integrating internet of things functionalities in learning management systems. Education and Information Technologies, 25(4), 2699-2732.
Maphalala, M.C. & Adigun, O.T. 2021. Academics’ Experience of Implementing E-Learning in a South African Higher Education Institution. International Journal of Higher Education, 10(1):1-13.
Nakamura, M. 2017. The state of asynchronous education in Japan. Quarterly Review of Asynchronous Education, 18(3), 75–87.
Nigam, A. 2017. Adoption of Bharat Interface for Money (BHIM) Application using TAM Framework. International Journal of Computer Science & Management Studies, 11.
Simamora, R.M. 2020. The Challenges of online learning during the COVID-19-19 pandemic: An essay analysis of performing arts education students. Studies in Learning and Teaching, 1(2):86-103.
Venkatesh, V. & Davis, F.D. 2000. A Theoretical Extension of the Technology Acceptance Model: Four Longitudinal Field Studies. Management Science. 46(2), February, pp 186-204.