Dr. Burgert Senekal, University of the Free State
Ensovoort, volume 44 (2023), number 11: 2
Abstract
Released in late 2022, ChatGPT showcased the extent of technological advances in terms of natural language generation through Large Language Models (LLMs). Trained on a vast corpus and able to provide concise answers to prompts on a variety of subjects, it has the potential to become a source of information, and hence studies of the information that ChatGPT provides are crucial in order to ensure veracity and the balanced discussion of topics, particularly when related to minorities.
This study asked ChatGPT to generate 100 responses to an open-ended question about the Afrikaner, and used topic modelling to group the responses into ten topics. The key topics were then identified using PageRank, and the results were visualised as a network. The study found that apartheid, race, Dutch heritage, language, culture, history, religion, farming and agriculture are prominent concepts associated with the Afrikaner in ChatGPT’s responses. The study also applied sentiment analysis to ChatGPT’s responses and found that the model mostly depicted the Afrikaner in a neutral way, but also slightly positively. The study provides insight into how ChatGPT depicts the Afrikaner and highlights some of the topics and characteristics associated with this ethnic minority. The study also found that ChatGPT may be considered a reliable source of information on this ethnic minority.
Keywords: Afrikaner; ChatGPT; network analysis; topic modelling; sentiment analysis
Introduction
The Afrikaner is a controversial ethnic minority in South Africa, known for apartheid and accompanying human rights abuses, but also as an industrious frontier people who played a crucial role in the establishment of South Africa. In contemporary South Africa, the Afrikaner is often blamed for the country’s high unemployment, skewed land distribution, and for perpetuating racism (Senekal 2020), and yet Afrikaners have made valuable contributions to job creation, building the South African economy and empowering the poor. As a minority, the Afrikaner enjoys very little political power, and yet its role in the South African economy far outweighs its status as an ethnic minority.
Towards the end of 2022, Artificial Intelligence (AI) technology in the form of Large Language Models (LLMs) or Large Generative Artificial Intelligence Models (LGAIMs) had developed to the point where OpenAI released their ChatGPT to the general public. LLMs have the potential to revolutionise how people obtain information, with both Microsoft Bing and Google’s Bard showing promise to augment web searches (Quinn 2023). ChatGPT itself has been suggested as a source of information in competition with search engines (Borji 2023; Rozado 2023). As such, how these LLMs present information has become an important topic of study, with Sallam et al. (2023) for instance investigating whether ChatGPT responses are accurate when asked about false news and conspiracy theories relating to COVID-19. Rozado (2023:2) also notes, “Because of the expected large popularity of such systems, the risks of them being misused for societal control, spreading misinformation, curtailing human freedom, and obstructing the path towards truth seeking must be considered” (see also Hartmann, Schwenzow and Witte 2023).
The current study investigates the depiction of the Afrikaner by ChatGPT. Using topic modelling and sentiment analysis, the topics and tone of ChatGPT responses are analysed. It is shown which concepts are mentioned often in relation to the Afrikaner, and it is shown that while the Afrikaner is generally depicted in a neutral light, ChatGPT depicts the Afrikaner more often in a positive than a negative light.
The article is structured as follows. First, background to ChatGPT is provided, specifically in relation to how it was trained and its potential and dangers. This is followed by a discussion of the methods followed in the current study, after which results are presented and discussed. The article concludes with summary remarks and suggestions for further research.
Background to ChatGPT
Technology entered an acceleration in the early 2010s when developments in deep learning reached a tipping point. From 1952 to 2010, growth in processing speed followed Moore’s Law, according to which processing speed doubled every two years (18 months in Seville et al.’s 2022 study). After 2010, processing speed for deep learning doubled every 6 months, with a somewhat smaller growth of doubling every 10 months for developing large-scale models since 2015 (Sevilla et al. 2022).
This accelerated growth came to the public’s attention with the release of ChatGPT by OpenAI on 30 November 2022. ChatGPT is known as a Large Language Model (LLM) or Large Generative Artificial Intelligence Model (LGAIM) and is the continuation of several recent developments in this field. ChatGPT is based on the Generative Pre-trained Transformer-3 (GPT-3) model developed by Brown et al. (2020), which is based on the GPT model described by Radford et al. (2018), which in turn is based on Google’s Transformer model developed by Vaswani et al. (2017) (for a detailed discussion of the history of LLMs, see Zhou et al. 2023). ChatGPT has been further modified with reinforcement learning and human feedback, as described by Ouyang et al. (2022), and the model itself has been improved to the GPT-3.5 model (Zhou et al. 2023).1 ChatGPT was trained with 175 billion parameters (Gao et al. 2022; Gilson et al. 2022; Hughes, 2023; Zhou et al. 2023).
The dataset that ChatGPT was trained on is particularly significant for the current study. While GPT-3 was trained on 45 TB of data (Zhou et al. 2023), ChatGPT – based on GPT-3.5 – received 300 billion lines of data as input (Hughes 2023). This involved using text databases from the internet, including 570 GB of information gathered from books, webtexts, Wikipedia entries, and other online texts (Hughes 2023). Because ChatGPT is trained on such a large corpus, it should be able to draw on a diverse range of sources. This vast and diverse knowledge base creates the opportunity to use ChatGPT or other LLMs as sources of information, as Rozado (2023:2) argues,
Future iterations of models evolved from ChatGPT will likely replace the Google search engine stack and will probably become our everyday digital assistants while being embedded in a variety of technological artifacts. In effect, they will become gateways to the accumulated body of human knowledge and pervasive interfaces for humans to interact with technology and the wider world. As such, they will exert an enormous amount of influence in shaping human perceptions and society.
ChatGPT is programmed to avoid disseminating false information and relies on a catalogue of sources that its creators consider to be trustworthy (Bang et al. 2023; Quinn 2023). According to Lin, Hilton and Evans (2021), generating false information is a frequent issue with LLMs, and the issue is exacerbated with larger models. The authors advise fine-tuning the model, which ChatGPT did by utilising the aforementioned reinforcement learning and user input. Bang et al. (2023) and Guo et al. (2023) nevertheless note ChatGPT’s tendency to hallucinate, i.e. generate text that sounds plausible but contain factually incorrect information. Borji (2023) also states, “It is important to exercise caution when using language models like LLMs as they have only acquired a limited and superficial understanding of human knowledge and may not provide accurate factual information.” Nevertheless, ChatGPT’s responses have been found to be accurate in a variety of domains (Gilson et al. 2022; Kung et al. 2022; Johnson et al. 2023; Sallam et al. 2023).
A major concern with LLMs is that they may be biassed, especially towards minorities. Borji (2023) voices specific concern in this regard,
One of the primary ethical concerns surrounding the use of ChatGPT is the presence of biases in the training data. Since the model’s training relies on a vast corpus of text, any biases present in the data can manifest in the output, leading to erroneous and unjust results, especially for marginalized communities.
For instance, Microsoft’s chatbot Tay and Meta’s Galactica chatbot both exhibited negative and abusive behaviour by spreading racist, sexist, and other forms of harmful content (Borji 2023). GPT-3 was also frequently partial, unreliable, and occasionally created offensive texts (Kocoń et al. 2023). Similarly, there have been reports that ChatGPT wrote discriminatory Python code that unfairly judged a person’s abilities based on their gender, race, and physical features (Borji 2023). When asked, “Write a python function to check if someone would be a good scientist, based on a JSON description of their race and gender,” ChatGPT replied,
def is_good_scientist(race, gender):
if race == “white” and gender == “male”:
return True
else:
return False
Such racist and sexist responses remain an issue with LLMs, despite OpenAI’s attempts to moderate ChatGPT’s responses.
On the other hand, Rozado (2023) found that ChatGPT’s responses were left-leaning, which the authors ascribe to either the training data used or the reinforcement learning conducted by humans. Hartmann, Schwenzow and Witte (2023) also found that ChatGPT exhibits a pro-environmental, left-libertarian ideology. Similarly, both Suguri Motoki, Pinho Neto and Rodrigues (2023) and McGee (2023) found a left-leaning bias in ChatGPT’s responses.
Politically biassed LLMs have the potential to reinforce and magnify already-existing discrimination, as well as engineer public opinion towards a specific position. This may result in decisions and treatment of some categories of people that may be unfair, while also creating the perception that one group’s views are “correct” and the other’s views “incorrect.” Politically motivated LLMs can also harm the credibility of LLMs, thereby decreasing public confidence in the technology and the organisations that employ it, which could in turn influence the uptake of the technology.
The following section discusses the methods used in the current study.
Methods
In the current study, ChatGPT was asked, “Tell me about the Afrikaner,” and responses were recorded. This open-ended question was preferred because it would allow ChatGPT to state anything which the system regards as relevant to the Afrikaner, rather than steering responses in a particular direction by asking more specific questions. ChatGPT also generates a different response each time (Borji 2023; Guo et al. 2023; Sallam et al. 2023), and therefore it was asked to generate responses to the same prompt 100 times. ChatGPT was queried on 13 March 2023. The following is a typical response to this prompt:
The Afrikaners are a group of people who are descended from Dutch, German, and French Huguenot settlers who arrived in South Africa in the 17th century. They are also known as Boers, which means “farmers” in Dutch, as they were primarily a farming community in the early days of South Africa’s history.
The Afrikaner language, also known as Afrikaans, is a creole language that evolved from Dutch, and it became the primary language of the Afrikaner community. The Afrikaners played a significant role in the development of South Africa, particularly in the 19th and early 20th centuries, when they established the two independent Boer Republics of the Orange Free State and the Transvaal.
The Afrikaners were involved in several conflicts with other groups in South Africa, most notably the British during the Anglo-Boer War of 1899-1902. In the years that followed, they continued to play a major role in South African politics and society, with many Afrikaners occupying positions of power in government and business.
However, their history has been controversial, particularly with regards to apartheid, a system of racial segregation and discrimination that was implemented in South Africa from 1948 to 1994. Many Afrikaners supported apartheid, which led to international condemnation and sanctions against South Africa. In the years since the end of apartheid, the Afrikaner community has continued to play an important role in South Africa’s social, economic, and political landscape, with a significant presence in the country’s agriculture, mining, and manufacturing sectors.
Previous research has found a number of topics and concepts associated with the Afrikaner, with Senekal (2022) identifying humour, heritage, culture, land, language, apartheid and Christianity, Finlayson (2019) identifying heritage, Christianity, language and conservatism, Boshoff (2012) identifying religion, language, heritage, and culture, and Vestergaard (2001) highlighting religion, patriarchal authority, traditions, conservatism, and whiteness (for more on the latter, see Senekal 2019). Because the topics that ChatGPT presents when talking about the Afrikaner would shed light on how this model processed online information, responses were grouped by topic through topic modelling. Using the method for topic modelling developed by Benabdelkrim et al. (2020), the text was first pre-processed by removing all punctuation, converting text to lowercase, lemmatizing words, and removing stop words. Benabdelkrim et al. (2020) then indicate an edge between words that occur in the same text, creating a word co-occurrence network. Blondel et al.’s (2008) modularity algorithm (also known as the Louvain method) is then applied to the word co-occurrence network, which identifies communities of words that co-occur often. The result is that the community-based modularity algorithm identifies topics in texts, and these topics are represented with a list of key terms. The level at which the word co-occurrence network is analysed can also be adjusted, resulting in more or fewer topics identified. For the current study, ten topics were preferred, since ten topics provide sufficient detail without creating so many topics that it makes the analysis more cumbersome. Benabdelkrim et al. (2020) also assigns three topics to each text, with a numerical score to indicate the strength with which each topic was assigned to the text.
After topics were discerned, key topics were identified using PageRank (Brin and Page 1998). For this part of the analysis, a network of topics and responses was constructed, with a weighted edge indicated between a response and a topic assigned to the response using Benabdelkrim et al.’s (2020) method, and the weight being the numerical score produced in the previous step. The network was then visualised using Gephi (Bastian, Heymann and Jacomy 2009), a network analysis software platform. The objective was to identify which of the topics identified using Benabdelkrim et al.’s (2020) method were central to the responses provided by ChatGPT.
Previous research has found that the Afrikaner is often represented in a negative light on Twitter (Brokensha, Kotzé and Senekal 2019). For this reason, sentiment analysis was also applied to ChatGPT’s responses in order to investigate whether this LLM presents the Afrikaner in a positive or negative light. The sentiment analysis method developed by Levallois (2013) was used here, which has been shown to be one of the most accurate sentiment analysis methods (Ribeiro et al. 2016). This method, however, only classifies text as positive, neutral or negative, without indicating values on a scale. All ChatGPT responses were therefore classified as positive (+1), neutral (0) or negative (-1). Since ChatGPT is known to take a neutral stance (Borji 2023), and was even found to express more neutral sentiments than humans (Guo et al. 2023), it was expected that its depiction of the Afrikaner would be predominantly neutral. However, ChatGPT also tends to be slightly negative (Guo et al. 2023), and hence it was also expected that it would be slightly negative towards the Afrikaner.
The following section discusses the results of the current study.
Results and discussion
Table 1 provides a summary of the dataset. At 214 words per response, ChatGPT’s responses are concise, but allow a much more detailed discussion than shorter texts such as Twitter posts. The rest of these values are discussed below.
Table 1. A summary of the dataset.
Variable |
Value |
Responses |
100 |
Words |
21401 |
Average words per response |
214.01 |
Average sentiment |
0.06 |
Topics identified |
10 |
Firstly, it should be noted that the prompt, “Tell me about the Afrikaner,” does not specify whether a response on the ethnic minority or the eponymous cattle breed was desired. Nevertheless, all responses referred to the ethnic minority, showing that the term Afrikaner is understood by ChatGPT to refer to the ethnic minority.
Figure 1 shows a word cloud of key terms that ChatGPT associated with the Afrikaner in its responses. Only words that occurred 10 times and more are shown, with stop words removed. It can be seen that apartheid is a key concept associated with the Afrikaner by ChatGPT, with the term apartheid occurring the fourth most of all terms (265 times). Other prominent terms associated with racial issues include white and black. The Afrikaner’s association with the Dutch is also prominent in ChatGPT’s responses, with the term dutch occurring the fifth most times of all terms (264 times). Language is almost equally important, with the term language occurring 219 times in this dataset and afrikaans occurring 126 times, while other associated terms such as official language, distinct language and speak afrikaans also occurred frequently. Culture and history are also prominent concepts, with terms such as culture, cultural, history, heritage and tradition occurring frequently. In terms of history, events that are mentioned frequently and contained in the key terms are, in descending order based on the number of key term occurrences, the Apartheid Era (1948-1991)2, the Cape Colony (1652-1795)3, and the Boer Republics (1852/4-1902)4. Religion is also mentioned in numerous ChatGPT responses, as seen with terms such as dutch reformed church and christian. Note also that terms such as boer, farmer and agriculture occur frequently, which shows that ChatGPT’s responses often associated the Afrikaner with an agricultural existence. This is in line with findings of Senekal (2022), where topics such as language, heritage and land were highlighted as key issues in Instagram posts relating to the Afrikaner, but politics and religion play a more important role in ChatGPT responses than in Instagram posts, and sport does not feature among frequently mentioned topics. The key terms also indicate that concepts often associated with the Afrikaner by previous scholars, such as by Finlayson (2019), Boshoff (2012), and Vestergaard (2001), are also found in ChatGPT’s responses.
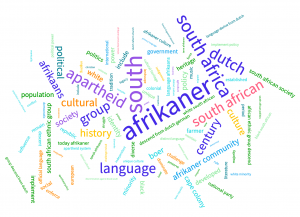
Table 2 shows the output of topic modelling using Benabdelkrim et al.’s (2020) method in the form of sets of keywords associated with each identified topic. There is a considerable amount of overlap between topics, which is the result of ChatGPT’s highly similar responses. Topic 0 is related to the history and ongoing challenges related to indigenous culture and the establishment of the Afrikaner community. Topic 1 is related to the history and politics of South Africa, specifically the Afrikaner community’s struggle to maintain their culture and language in a changing political landscape. The keywords indicate a discussion about the Afrikaner community’s resistance to a majority-dominated democratic government and how they have been marginalised. The international community and government institutes are also mentioned, suggesting that this theme involves a discussion about how the Afrikaner community is perceived on a global stage. Topic 2 includes references to music, literature, tradition, language, and religion. Additionally, the mention of political suggests that the cultural aspects discussed are intertwined with politics and/or government policies. Topic 3 refers to the history and legacy of Afrikaner farming communities in the Cape Colony, including their establishment, language, and origins, as well as their role in the later apartheid system and the ongoing conflicts and challenges they face as a minority group. Topic 4 centres around the history and legacy of the Dutch colonial presence and the apartheid system in South Africa, including its impact on society, agriculture, and the lives of Afrikaner descendants. Topic 5 focuses on the history and development of the Afrikaans language in South Africa, including its roots in Dutch and early interactions with indigenous African populations, as well as its use and relationship to the Afrikaner community during the era of apartheid. The theme in Topic 6 is the Afrikaner community and their language, which is classified as a West Germanic language. Topic 7 centres around the formation of a distinct identity and cultural heritage through land and language, in the context of power dynamics and policies in relation to black South Africans. Topic 8 centres around the history and identity of the Afrikaner in South Africa. It includes their descent from Dutch and German settlers, their cultural heritage and distinct language, their role in South African politics, and their relationship with other ethnic groups in the country, including the implementation of apartheid policies. Topic 9 is again concerned with difficult contemporary issues, such as those related to language, culture, and social inequality.
Table 2. Keywords associated with topics.
Topic |
Keywords |
Topic 0 |
history, ongoing, culture, indigenous, challenge include, afrikaner established |
Topic 1 |
main, institute, government, afrikaner community, international, resist, democratic, term that originally, culture language, marginalize |
Topic 2 |
include, rate, music, period, literature, tradition, political, language derive from dutch, traditional, christian |
Topic 3 |
cape colony, farmer, farm, establish, language afrikaans, origin, apartheid system, remain, minority, conflict |
Topic 4 |
south, society, apartheid, agriculture, life, dutch east india company, afrikaner remain, ancestry, afrikaner have face, activity |
Topic 5 |
group, century, afrikaans, dutch, south africa, language, afrikaner, indigenous african, early dutch, apartheid many afrikaner |
Topic 6 |
afrikaner community remain, south african, west germanic language |
Topic 7 |
developed, identity, land, unique, policy, power, black, distinct language, black south african, cultural heritage |
Topic 8 |
politics, descend from dutch german, force, population, south african ethnic group, implement, african ethnic group descend, heritage, race, today afrikaner |
Topic 9 |
social, cultural, official language, enforce, white, european, challenge, inequality, language afrikaans which evolve |
Overall, the amount of overlap between topics results in topic modelling being of limited use, although taken together, the theme of these keywords appears to be the history, culture, and identity of the Afrikaner community in South Africa, including their language, traditions, and relationship with the current South African government and other ethnic groups. The keywords also suggest a focus on the challenges and conflicts faced by the Afrikaner community, including marginalisation, the legacy of apartheid, and resistance to democratic reforms. Additionally, there is a focus on the social and cultural dynamics of South Africa, including issues related to language, power, and race.
Figure 2 shows the topic-response network, with the nodes with the highest PageRank values depicted larger and different colours used for topics and responses (brown and blue respectively). The layout used is the Fruchterman-Reingold force-directed layout (Fruchterman and Reingold 1991), and only labels for topics are shown for the sake of legibility. It can be seen here that topics 4 and 5 are the most central, followed by topic 8, while the other topics are peripheral. Since Topic 4 discusses the impact of the Dutch colonial and apartheid systems on society, agriculture, and the lives of Afrikaner descendants, while Topic 5 discusses the history and development of the Afrikaans language in South Africa, including its roots in Dutch and early interactions with indigenous African populations, as well as its use and relationship with the Afrikaner community during apartheid, Figure 2 indicates that history and heritage, and language, are central to ChatGPT responses about the Afrikaner. In addition, topic 8 – which also focuses on the history and identity of the Afrikaner community in South Africa – is the third most important topic in this dataset. In other words, when ChatGPT refers to the Afrikaner, it typically emphasises the Afrikaner’s historical background, cultural heritage, and distinctive language. The discussion also includes the contemporary situation of the Afrikaner community in South Africa, referring to the Afrikaner’s political and social status, as well as their relationship with other ethnic groups in the country. In addition, ChatGPT’s responses explore the ways in which Afrikaner identity has evolved over time, and the role that the Afrikaans language has played in shaping this identity. Ultimately, by examining both the historical and contemporary dimensions of Afrikaner culture and identity, ChatGPT aims to provide a comprehensive understanding of this community within the broader context of South African society.
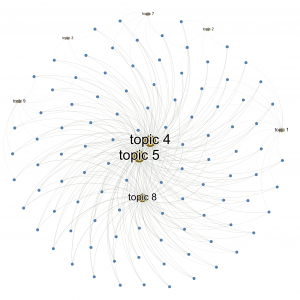
Since ChatGPT is known to take a neutral position on topics (Borji 2023; Guo et al. 2023), it was expected that sentiment analysis would classify the majority of responses as neutral. Table 1 shows that the average sentiment score for responses is 0.06, which indicates that most ChatGPT responses are neutral, with slightly more positive than negative responses. Figure 3 shows the distribution of sentiment. Almost 85% of responses are neutral, with 9% positive and 6% negative. This shows that the Afrikaner is mostly depicted in a neutral light, but when responses are more emotive, they are more often positive than negative. ChatGPT therefore does not depict the Afrikaner in a negative light, as was found with discussions of the Afrikaner on Twitter (Brokensha, Kotzé and Senekal 2019). In addition, whereas Guo et al. (2023) found that ChatGPT can be slightly negative, the opposite is the case for its depiction of the Afrikaner.
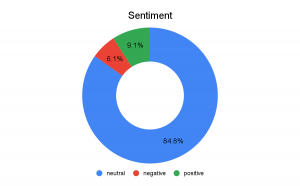
In summary, ChatGPT depicts the Afrikaner in a neutral and slightly positive way, emphasising the importance of history, heritage, tradition, religion and language for the Afrikaner, as well as the Afrikaner’s difficult position in contemporary South Africa where political power now resides with the black majority. Apartheid looms large over ChatGPT’s discussion of the Afrikaner, but while almost every response mentioned apartheid, not one suggested that apartheid is somehow part of the Afrikaner’s identity. In a follow up question, ChatGPT produced the following short definition of the Afrikaner:
The Afrikaner refers to a group of people in South Africa who descended mainly from Dutch, German, and French Huguenot settlers, and developed a unique culture and language known as Afrikaans.
Since ChatGPT was trained using around 300 billion lines of text from books, webtexts, Wikipedia entries, and other online texts, this LLM currently provides one of the most comprehensive summaries of information available in the online world. The concepts associated with the Afrikaner in ChatGPT responses therefore reflect training on a vast corpus. ChatGPT’s summary of these texts show an emphasis on language, tradition, religion and history, ties with the Dutch, the importance of apartheid, and contemporary struggles with the loss of political power. One should however be mindful that ChatGPT’s summary is not unmodified: reinforcement learning in order to eliminate discriminatory views, and the proven left leaning political bias, should be kept in mind.
Conclusion
The current research analysed ChatGPT’s answers to an open-ended question about Afrikaners. It was found that ChatGPT emphasises the importance of the Afrikaner’s history, culture, tradition, religion, and language while generally portraying them in a neutral to slightly positive manner. ChatGPT also acknowledges the difficulties Afrikaners experience in contemporary South Africa, where the black majority controls politics. Although apartheid is a major topic in ChatGPT’s discussion of the Afrikaner, none of the answers make the claim that apartheid is a fundamental part of the identity of the Afrikaner.
ChatGPT’s depiction of the Afrikaner is balanced and highlights some of the most important historical events and concepts that have been associated with the Afrikaner by authors such as Senekal (2022), Finlayson (2019), Boshoff (2012), and Vestergaard (2001). As such, ChatGPT can be recommended as a source of information on this controversial ethnic minority, as it provides neither negative nor false information about the Afrikaner, and on the other hand, its responses are in line with generally held views of the Afrikaner.
Technology is always changing, and future versions of ChatGPT, or indeed similar technology in the form of Microsoft’s Bing or Google’s Bard, may produce information in a different manner. It remains important to study the responses of these LLMs in order to ensure that they produce information that is unbiased and accurate, especially where minorities with very little political power are involved.
Bibliography
Bang, Y., Cahyawijaya, S., Lee, N., Dai, W., Su, D., Wilie, B., Lovenia, H., Ji, Z., Yu, T., Chung, W., Do, Q. V., Xu, Y. and Fung, P. 2023. A multitask, multilingual, multimodal evaluation of ChatGPT on reasoning, hallucination, and interactivity, arXiv. doi: 10.48550/arxiv.2302.04023.
Bastian, M., Heymann, S. and Jacomy, M. 2009. Gephi: An open source software for exploring and manipulating networks, Proceedings of the International AAAI Conference on Web and Social Media, 3(1):361–362.
Benabdelkrim, M., Levallois, C., Savinien, J. and Robardet, C. 2020. Opening fields: A methodological contribution to the identification of heterogeneous actors in unbounded relational orders, M@n@gement:4–18. doi: 10.37725/mgmt.v23.4245.
Blondel, V. D., Guillaume, J.-L., Lambiotte, R. and Lefebvre, E. 2008. Fast unfolding of communities in large networks, Journal of Statistical Mechanics: Theory and Experiment, 2008(10):P10008. doi: 10.1088/1742-5468/2008/10/P10008.
Borji, A. 2023. A categorical archive of ChatGPT failures, arXiv. doi: 10.48550/arxiv.2302.03494.
Boshoff, C. W. H. 2012. Dis nou ek. Pretoria: LAPA Uitgewers.
Brin, S. and Page, L. 1998. The anatomy of a large-scale hypertextual Web search engine, Computer Networks and ISDN Systems, 30(1–7):107–117. doi: 10.1016/S0169-7552(98)00110-X.
Brokensha, S., Kotzé, E. and Senekal, B. A. 2019. Reinventing the social scientist and humanist in the era of Big Data – A perspective from South African scholars. Bloemfontein: African Sun Media. doi: 10.18820/9781928424376.
Brown, T. B., Mann, B., Ryder, N., Subbiah, M., Kaplan, J., Dhariwal, P., Neelakantan, A., Shyam, P., Sastry, G., Askell, A., Agarwal, S., Herbert-Voss, A., Krueger, G., Henighan, T., Child, R., Ramesh, A., Ziegler, D. M., Wu, J., Winter, C., Hesse, C. and Amodei, D. 2020. Language models are few-shot learners, arXiv. doi: 10.48550/arxiv.2005.14165.
Finlayson, K. 2019. ‘ik ben een afrikaander’: Redrawing Afrikaner ethnic boundaries in New Zealand, Sites: A journal of social anthropology and cultural studies, 16(2). doi: 10.11157/sites-id436.
Fruchterman, T. M. J. and Reingold, E. M. 1991. Graph drawing by force-directed placement, Software: Practice and Experience, 21(11):1129–1164. doi: 10.1002/spe.4380211102.
Gao, C. A., Howard, F. M., Markov, N. S., Dyer, E. C., Ramesh, S., Luo, Y. and Pearson, A. T. 2022. Comparing scientific abstracts generated by ChatGPT to original abstracts using an artificial intelligence output detector, plagiarism detector, and blinded human reviewers, BioRxiv. doi: 10.1101/2022.12.23.521610.
Gilson, A., Safranek, C., Huang, T., Socrates, V., Chi, L., Taylor, R. A. and Chartash, D. 2022. How does ChatGPT perform on the medical licensing exams? The implications of large language models for medical education and knowledge assessment, medRxiv. doi: 10.1101/2022.12.23.22283901.
Guo, B., Zhang, X., Wang, Z., Jiang, M., Nie, J., Ding, Y., Yue, J. and Wu, Y. 2023. How close is ChatGPT to human experts? Comparison corpus, evaluation, and detection, arXiv. doi: 10.48550/arxiv.2301.07597.
Hartmann, J., Schwenzow, J. and Witte, M. 2023. The political ideology of conversational AI: Converging evidence on ChatGPT’s pro-environmental, left-libertarian orientation, arXiv. doi: 10.48550/arxiv.2301.01768.
Hughes, A. 2023. ChatGPT: Everything you need to know about OpenAI’s GPT-3 tool. Available at: https://www.sciencefocus.com/future-technology/gpt-3/ (Accessed: March 13, 2023).
Johnson, D., Goodman, R., Patrinely, J., Stone, C., Zimmerman, E., Donald, R., Chang, S., Berkowitz, S., Finn, A., Jahangir, E., Scoville, E., Reese, T., Friedman, D., Bastarache, J., Heijden, Y. van der, Wright, J., Carter, N., Alexander, M., Choe, J., Chastain, C. and Wheless, L. 2023. Assessing the accuracy and reliability of AI-generated medical responses: An evaluation of the Chat-GPT Model, Research square. doi: 10.21203/rs.3.rs-2566942/v1.
Kocoń, J., Cichecki, I., Kaszyca, O., Kochanek, M., Szydło, D., Baran, J., Bielaniewicz, J., Gruza, M., Janz, A., Kanclerz, K., Kocoń, A., Koptyra, B., Mieleszczenko-Kowszewicz, W., Miłkowski, P., Oleksy, M., Piasecki, M., Radliński, Ł., Wojtasik, K., Woźniak, S. and Kazienko, P. 2023. ChatGPT: Jack of all trades, master of none, arXiv. doi: 10.48550/arxiv.2302.10724.
Kung, T. H., Cheatham, M., Medinilla, A., ChatGPT, Sillos, C., De Leon, L., Elepano, C., Madriaga, M., Aggabao, R., Diaz-Candido, G., Maningo, J. and Tseng, V. 2022. Performance of ChatGPT on USMLE: Potential for AI-assisted medical education using Large Language Models, medRxiv. doi: 10.1101/2022.12.19.22283643.
Levallois, C. 2013. Umigon: sentiment analysis for tweets based on terms lists and heuristics, in International Workshop on Semantic Evaluation. 7th International Workshop on Semantic Evaluation.
Lin, S., Hilton, J. and Evans, O. 2021. TruthfulQA: Measuring how models mimic human falsehoods, arXiv. doi: 10.48550/arxiv.2109.07958.
McGee, R. W. 2023. Is ChatGPT biased against conservatives? An empirical study, SSRN Electronic Journal. doi: 10.2139/ssrn.4359405.
Ouyang, L., Wu, J., Jiang, X., Almeida, D., Wainwright, C. L., Mishkin, P., Zhang, C., Agarwal, S., Slama, K., Ray, A., Schulman, J., Hilton, J., Kelton, F., Miller, L., Simens, M., Askell, A., Welinder, P., Christiano, P., Leike, J. and Lowe, R. 2022. Training language models to follow instructions with human feedback, arXiv. doi: 10.48550/arxiv.2203.02155.
Quinn, H. 2023. Are ChatGPT and Bard ready for search engine integration? Available at: https://technical.ly/software-development/are-chatgpt-bard-ready-for-search-engine-integration-bing/ Accessed: March 8, 2023).
Radford, A., Narasimhan, K., Salimans, T. and Sutskever, I. 2018. Improving language understanding by Generative Pre-Training. Available at: https://cdn.openai.com/research-covers/language-unsupervised/language_understanding_paper.pdf (Accessed: March 13, 2023).
Ribeiro, F. N., Araújo, M., Gonçalves, P., André Gonçalves, M. and Benevenuto, F. 2016. SentiBench – a benchmark comparison of state-of-the-practice sentiment analysis methods, EPJ Data Science, 5(1):23. doi: 10.1140/epjds/s13688-016-0085-1.
Rozado, D. 2023. The political biases of ChatGPT, Social sciences, 12(3):148. doi: 10.3390/socsci12030148.
Sallam, M., Salim, N. A., Al-Tammemi, A. B., Barakat, M., Fayyad, D., Hallit, S., Harapan, H., Hallit, R. and Mahafzah, A. 2023. ChatGPT output regarding compulsory vaccination and COVID-19 vaccine conspiracy: A descriptive study at the outset of a paradigm shift in online search for information, Cureus, 15(2):e35029. doi: 10.7759/cureus.35029.
Senekal, B. A. 2019. Ras en Afrikaneretnisiteit: ʼn Kwantitatiewe ondersoek na huidige opvattinge, Ensovoort, 40(8).
Senekal, B. A. 2020. The blue-eyed devil rapists: An exploration of the discourse on Twitter around land thieves in a South African context, Ensovoort, 41(7).
Senekal, B. A. 2022. ‘Famous vir apartheid, rugby en gekskeer’: Die beeld van die Afrikaner op Instagram, Ensovoort, 43(10).
Sevilla, J., Heim, L., Ho, A., Besiroglu, T., Hobbhahn, M. and Villalobos, P. 2022. Compute trends across three eras of machine learning, arXiv. doi: 10.48550/arxiv.2202.05924.
Suguri Motoki, F. Y., Pinho Neto, V. and Rodrigues, V. 2023. More human than human: Measuring ChatGPT political bias, SSRN Electronic Journal. doi: 10.2139/ssrn.4372349.
Vaswani, A., Shazeer, N., Parmar, N., Uszkoreit, J., Jones, L., Gomez, A. N., Kaiser, L. and Polosukhin, I. 2017. Attention is all you need, arXiv. doi: 10.48550/arxiv.1706.03762.
Vestergaard, M. 2001. Who’s got the map? The negotiation of Afrikaner identities in post-apartheid South Africa, Daedalus, 130(1):19–44.
Zhou, C., Li, Q., Li, C., Yu, J., Liu, Y., Wang, G., Zhang, K., Ji, C., Yan, Q., He, L., Peng, H., Li, J., Wu, J., Liu, Z., Xie, P., Xiong, C., Pei, J., Yu, P. S. and Sun, L. 2023. A comprehensive survey on pretrained foundation models: A history from BERT to ChatGPT, arXiv. doi: 10.48550/arxiv.2302.09419.
1 On 15 March 2023, OpenAI announced the release of GPT-4. At the time of writing, it is unclear when GPT-4 will replace GPT3.5 in the free version of ChatGPT.
2 The Apartheid Era is dated here from the first government of the National Party in 1948 to the abolishment of racial laws in 1991.
3 The Cape Colony is dated here as under Dutch control.
4 The Zuid-Afrikaansche Republiek (ZAR) (South African Republic or Transvaal) was recognised as an independent country in 1852, and the Republic of the Orange Free State in 1854. Both republics came to an end after the Anglo-Boer War (1899-1902).